Applying Data Science to Improve Business Decision-Making
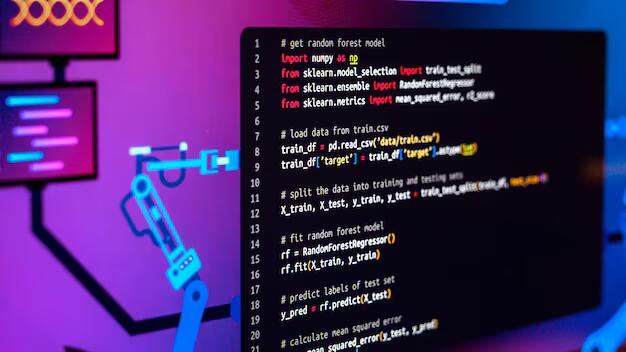
Understanding the Role of Data Science in Business Dynamics
- Identifying Trends: Businesses can harness advanced algorithms to predict consumer behavior shifts. For instance, a retail chain boosted sales by 20% by analyzing purchasing data to tailor personalized promotions.
- Optimizing Operations: Through predictive maintenance, manufacturers like GE reduce downtime by 30%, enhancing efficiency and cutting costs.
- Enhancing Customer Experience: Netflix’s recommendation engine, driven by data science, retains 93% of subscribers by offering tailored content suggestions.
- Risk Management: Financial firms utilize data science to detect fraud in real-time, reducing loss percentages significantly. For example, one bank decreased fraud instances by 25% using anomaly detection models.
Transformative Tools and Techniques in Data Science
Unlocking competitive advantage with transformative data science tools and techniques can be a game-changer for businesses. First and foremost, machine learning algorithms refine decision-making by sifting through massive datasets to identify patterns that are not intuitive to humans. For instance, major logistics companies use dynamic routing algorithms that have reduced delivery time by as much as 20% and shaved off fuel costs.
Advanced data visualization tools include Tableau and Power BI that enable the decision-maker to interact dynamically with data by showing insights through intuitive dashboards and real-time analytics. That also gives executives agility in strategy adjustments regarding the very latest market trends.
This leads to better customer understanding and gives deeper insights into the feedback from their customers and social media sentiments, thus enhancing product development and marketing strategies. With NLP, a cosmetic brand aligned product launches with the customer sentiment, leading to a 15% sales increase.
Moreover, cloud-based platforms provide scalable data processing, enabling small businesses to harness big data without hefty infrastructure investments. By adopting these sophisticated tools, businesses can sharpen strategic focus, drive innovation, and secure a robust foothold in today’s data-driven economy.
Leveraging Predictive Analytics for Strategic Decisions
Predictive analytics revolutionizes strategic decision-making by identifying patterns in historical data to predict future outcomes. For example, Starbucks enhanced its store placement strategy by using predictive models, leading to a 15% increase in foot traffic. Using customer transaction data, companies can predict demand spikes, enabling them to manage inventories precisely and minimize costs while maximizing sales opportunities.
Predictive analytics helps create campaigns in this dynamically changing world of marketing with laser-like precision. Example: A telecommunications company used targeted offers, extracted from the results of a predictive analytics model, to increase customer retention by 20%. Similarly, HR departments are able to predict workforce trends, using predictive analytics to proactively address skill gaps and improve employee satisfaction.
Moreover, businesses that merge predictive analytics with real-time data have a strategic advantage; they adapt to market shifts with speed and ensure their decisions are always based on the latest intelligence.
Case Studies from the Real World: Success Stories of Business with Data
Pricing to Perfection: With more than one million transaction records analyzed using machine learning models, a global retailer nailed an 8% increase in their profit margins. This real-world pricing strategy proved how effective and agile data-driven decisions can be.
Route Optimization of Airlines: By leveraging sophisticated predictive analytics, a leading airline cut fuel consumption by 12%. This optimization of flight routes cost-effectively reduced consumption, boosting eco-efficiency-a dual win for profitability and sustainability.
Healthcare Innovation: A hospital system applied data science to forecast the admission rates of patients, increasing the efficiency of resource utilization by 15%. The foresight helped ensure that staff levels were optimally managed to avoid long wait times and further enhanced the quality of care.
Challenges and Ethical Considerations in Data Application
Business decision-making with data science has quite a few challenges to go along with the ethical dilemmas in the landscape. Some of the major challenges revolve around the quality of the data itself. Poor data could very well equate to misguided insights and faulty decisions. A survey by Dimensional Research shows that 78% of businesses find inaccuracies in data reduce effective decision-making. Therefore, data integrity through rigorous validation is critical to ensure.
Other complications arise on ethical grounds, like algorithm biases, where the latest finding on racial and gender prejudices by a 2020 MIT study still seems to take a lead among generally used systems of facial recognition. Algorithms tainted with biases will be biased toward unjust decisions over specific groups and hence seriously impair trust in, and even corporate reputation of, your business. Such issues require the implementation of fair data practices: bias audits and diverse data training sets to ensure that all are treated equitably.
Another very serious ethical issue involves data privacy. With regulations like the GDPR and CCPA, businesses must navigate through increasingly complex compliance landscapes with severe penalties for non-compliance. Legal risk can be mitigated and consumer trust developed by developing transparent data policies and focusing on customer consent.
In return, the proactive identification of such challenges secures the businesses not only in operation but also fosters long-term strategic integrity and success.
The Road Ahead: Emerging Trends in Data Science for Businesses
As businesses forge on in the increasingly data-driven economy, a number of emerging trends in data science are going to redefine strategic decision-making. First among them is the integration of augmented analytics, which uses artificial intelligence to automate data preparation and insight discovery. According to a report by Gartner, by 2025, 75% of data analytics will be self-serve, enabling executives to get actionable insights without requiring broad technical skills.
Another fast-emerging trend is the integration of edge computing in data science. In this model, the data processing is performed locally near the source, thus reducing latency and bandwidth costs greatly. An example would be retail companies leveraging edge computing to process in-store video feeds to enable real-time inventory placement for improving customer experiences.
Besides that, more and more companies are working on ethical AI to earn consumers’ trust. A 2023 study by IBM reported that 85% of consumers would opt for companies that are ethically responsible. Transparency in AI models guarantees accountability and builds confidence among consumers.
Finally, the convergence of IoT and data science promises unprecedented possibilities. Companies capable of harnessing IoT data can anticipate operational disruptions, minimize downtime, and achieve a 30% improvement in predictive maintenance accuracy. These advancements position businesses to not only adapt but thrive in the future landscape.
Conclusion: Maximizing Business Potential through Data-Driven Insights
In the final analysis, data science is one of the key drivers behind modernizing business decision-making and providing a route toward high performance and competitive advantage. Identifying trends, optimizing operations, improving customer experience, and managing risks are the core capabilities of data science, thereby allowing businesses to convert large amounts of data into actionable insights. Real-life success stories, such as retail price optimization and efficiency in airline routes, reflect its transformative power.
Machine learning, predictive analytics, and advanced visualization tools integrated into the core enable businesses to make informed decisions faster and more accurately. However, ensuring data integrity and ethical concerns regarding algorithmic bias and privacy remain paramount. These can only be addressed through sound data validation and just practice for sustainable success.
With the continuously evolving landscape, emerging trends such as augmented analytics, edge computing, and the convergence of IoT promise much more in terms of efficiency and innovation. Businesses that embrace these move towards a resilient foothold in the data-driven economy, ensuring adaptability and thriving growth. In the end, effective leverage of data science will unlock new opportunities leading to strategic focus and measurable business growth.
Leave a Reply